Hashicorp Series Boundary
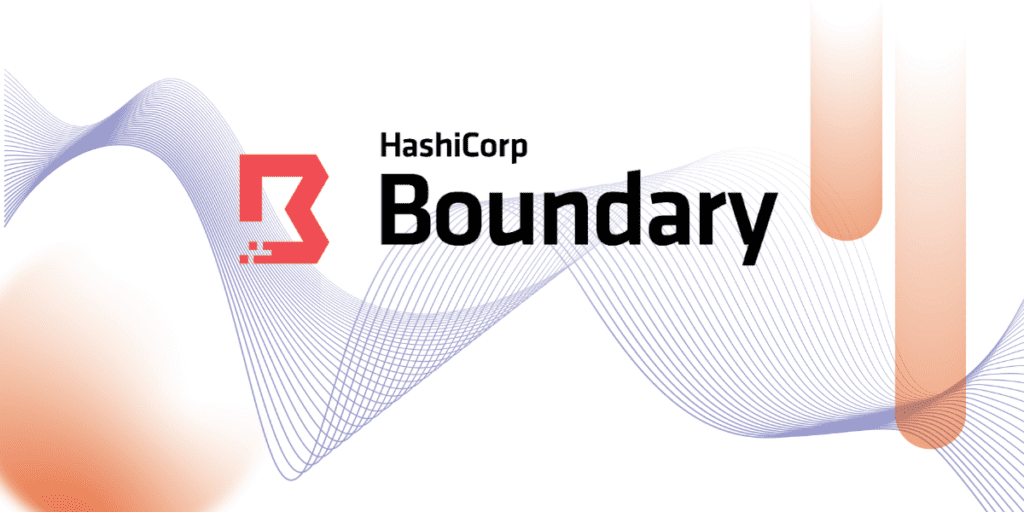
After the last HashiConf Digital, the Cloud Practice wants to present you one of the main innovations that were presented: Boundary. In this post we are going to discuss what offers this new tool, why it is interesting, what we have found and how we have tested it.
What is Hashicorp Boundary?
Hashicorp Boundary is, as themselves claim, a tool that allows access any system using identity as a fundamental piece. What does this really mean?
Traditionally, when a user acquires the permission to access a remote service, he or she also gets explicit permission to the network where the service resides. However, Boundary, following the minimum privilege principle, provides us with an identity-based system for users who need access to applications or machines. For example, it is an easy way of access to a server via SSH using ephemeral keys as authentication method.
This means that Boundary limits what resources you can connect to and also manages the different permissions and accesses to resources with an authentication.
It is especially interesting because in the future it will be marked by the strong integration that it will have with other Hashicorp tools, especially Vault for credentials management and audit capabilities.
In case you are curious, Hashicorp has released the source code of Boundary which you have available at Github and the official documentation can be read on their website:
boundaryproject.
How have we tested Boundary?
BBased on an example project from Hashicorp, we have developed a small proof of concept that deploys Boundary in a hybrid-cloud scenario in AWS and GCP. Although the reference architecture does not said nothing about this design, we wanted to complete the picture and
set up a small multi-cloud stage to see how this new product.
The final architecture in broad terms is:
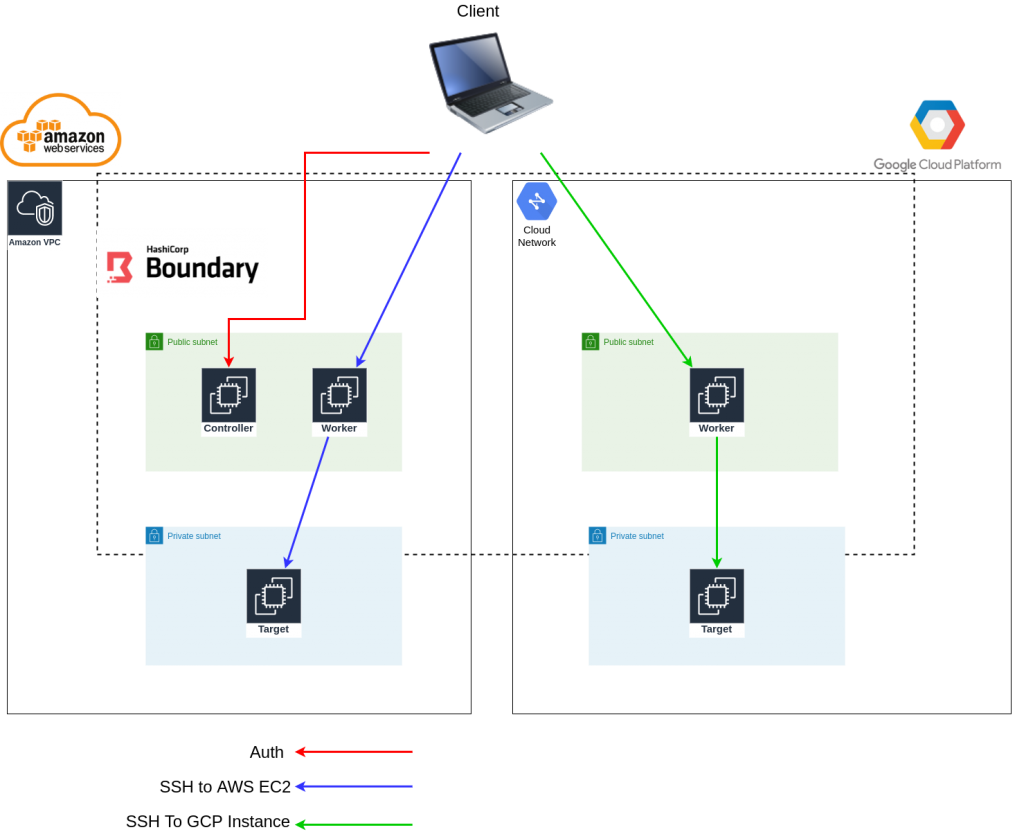
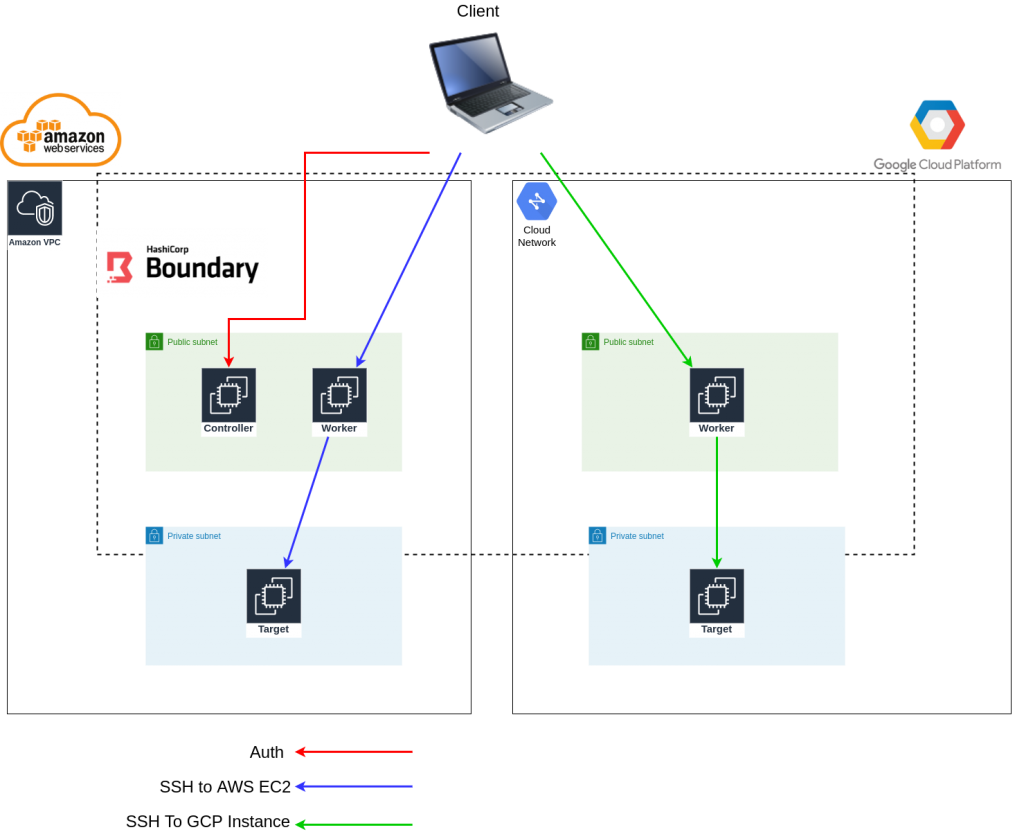
Once the infrastructure has been deployed and the application configured, we have tested connecting to the instances through SSH. All the source code is based on terraform 0.13 and you can find it in Bluetab-boundary-hybrid-architecture, where you will also find a detailed README that specifies the actions you have to follow to reproduce the environment, in particular:
- Authentication with your user (previously configured) in Boundary. To accomplish this, you have to set the Boundary controllers endpoint and execute the following command: boundary authenticate.
- Execute: boundary connect ssh with the required parameters to point to the selected target (this target represents one or more instances or endpoints)
In this particular scenario, the target is composed by two different machines:
one in AWS and one in GCP. If Boundary is not told which particular instance you want to access from that target, it will provide access to one of them randomly. Automatically, once you have selected the machine you want to access, Boundary will route the request to the appropriate worker, who has access to that machine.
What did we like?
- The ease of configuration. Boundary knows exactly which worker has to address the request taking into account which service or machine is being requested.
As the entire deployment (both infrastructure and application) has been done using terraform, the output of one deployment serves as the input of the other and everything is perfectly integrated. - It offers both graphic interface and CLI access. Despite being in a very early stage of development, the same binary includes (when configured as controller) a very clean graphical interface, in the same style as the rest of the Hashicorp tools. However, as not all functionality is currently implemented through the interface it is necessary to make configuration using the CLI.
What would we have liked to see?
- Integration with Vault and indentity providers (IdPs) is still in the roadmap and until next versions it is not sure that it will be included.
- The current management of the JWT token from the Boundary client to the control plane which involves installing a secret management tool.
What do we still need to test?
Considering the level of progress of the current product development, we would be missing for understanding and trying to:
- Access management by modifying policies for different users.
- Perform a deepest research on the components that manage resources (scopes, organizations, host sets, etc.)
Why do we think this product has great future?
Once the product has completed several phases in the roadmap that Hashicorp has established, it will greatly simplify resources access management through bastions in organizations. Access to instances can be managed simply by adding or modifying the permissions that a user has, without having to distribute ssh keys, perform manual operations on the machines, etc.
In summary, this product gives us a new way to manage access to different resources. Not only through SSH, but it will be a way to manage access through roles to machines, databases, portals, etc. minimizing the possible attack vector when permissions are given to contractors. In addition, it is presented as a free and open source tool, which will not only integrate very effectively if you have the Hashicorp ecosystem deployed, but will also work seamlessly without the rest of Hashicorp’s tools.
One More Thing…
We encountered a problem caused by the way in which the information about the network addresses of controllers and workers for subsequent communication was stored. After running our scenario with a workaround based on iptables we decided to open a issue on Github. In only one day, they solved the problem by updating their code. We downloaded the new version of the code, tested it and it worked perfectly. Point in favour for Hashicorp for the speed of response and the efficiency they demonstrated. In addition, recently it has been published a new release of Boundary, including this fix along with many other features Boundary v0.1.2.